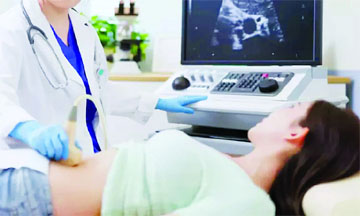
Scientists have combined machine learning with information on blood metabolites to develop a new test able to detect ovarian cancer with 93 per cent accuracy. For over three decades, a highly accurate early diagnostic test for ovarian cancer has eluded physicians.
Ovarian cancer is often referred to as the silent killer because the disease is typically asymptomatic when it first arises — and is usually not detected until later stages of development, when it is difficult to treat. According to John McDonald, Professor in the School of Biological Sciences at Georgia Institute of Technology, US, the new test’s accuracy is better in detecting ovarian cancer than existing tests for women clinically classified as normal, with a particular improvement in detecting early-stage ovarian disease in that cohort.
The new test, published in the online issue of the medical journal Gynecologic Oncology, uses a patient’s individual metabolic profile to assign a more accurate probability of the presence or absence of the disease. “This personalised, probabilistic approach to cancer diagnostics is more clinically informative and accurate than traditional binary (yes/no) tests,” McDonald said.
“It represents a promising new direction in the early detection of ovarian cancer, and perhaps other cancers as well.” McDonald said that while the average five-year survival rate for late-stage ovarian cancer patients, even after treatment, is around 31 per cent — but that if ovarian cancer is detected and treated early, the average five-year survival rate is more than 90 per cent. “Clearly, there is a tremendous need for an accurate early diagnostic test for this insidious disease,” McDonald said.
And although development of an early detection test for ovarian cancer has been vigorously pursued for more than three decades, the development of early, accurate diagnostic tests has proven elusive.
Because cancer begins on the molecular level, McDonald said, there are multiple possible pathways capable of leading to even the same cancer type.
“Because of this high-level molecular heterogeneity among patients, the identification of a single universal diagnostic biomarker of ovarian cancer has not been possible. “For this reason, we opted to use a branch of artificial intelligence — machine learning — to develop an alternative probabilistic approach to the challenge of ovarian cancer diagnostics.” The researchers developed their integrative approach by combining metabolomic profiles and machine learning-based classifiers to establish a diagnostic test with 93 per cent accuracy when tested on 564 women from Georgia, North Carolina, Philadelphia, and western Canada. Source: IANS
Be the first to comment